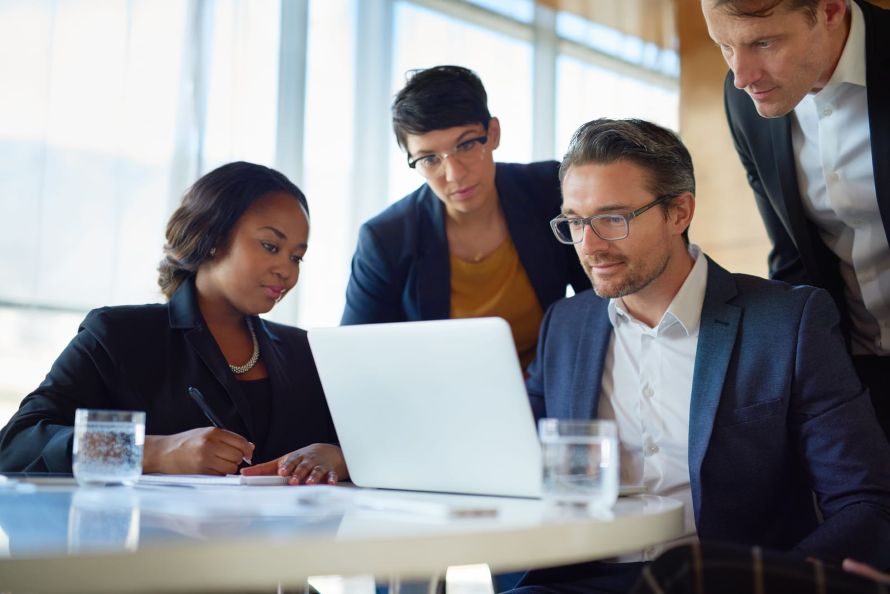
In a world where every fraudulent claim costs millions, artificial intelligence is reshaping the fight against deception in insurance. The integration of AI into fraud detection systems is turning traditional methods on their head, offering unprecedented accuracy and speed that insurers have never seen before. With rapid technological advances and a surge in data availability, AI is not only enhancing detection capabilities but also redefining the entire claims process.
Insurance fraud has long been a persistent challenge, draining resources and eroding trust in the industry. By leveraging machine learning, predictive analytics, and natural language processing, AI systems can sift through vast amounts of data in seconds, identifying patterns that would be nearly impossible for humans to catch. For instance, reputable resources like Forbes have highlighted the growing role of technology in combating fraud, illustrating the transformative impact on the industry.
This article explores how artificial intelligence is revolutionizing fraud detection in insurance claims, showcasing advanced technologies, practical benefits, and the insights of industry experts. Through a series of detailed sections, we examine the innovative techniques that drive these systems, the challenges they overcome, and the future trends that promise to further enhance fraud prevention. Let's embark on this journey into the heart of AI-driven fraud detection.
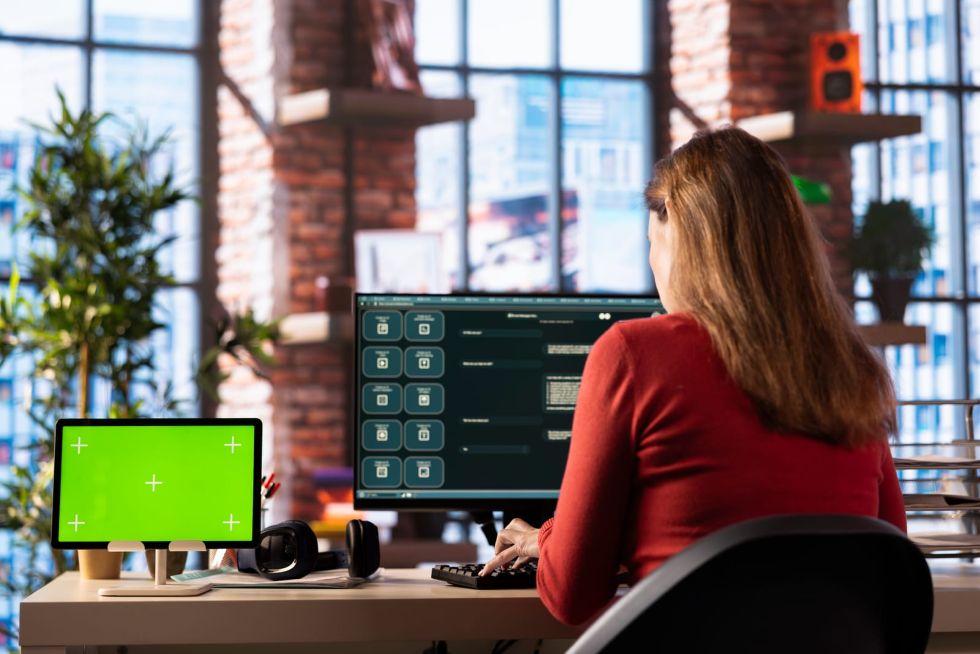
The Rise of AI in Fraud Detection
Artificial intelligence has rapidly become a game changer in the realm of insurance fraud detection. AI technologies are harnessing vast amounts of data and learning from patterns to predict and prevent fraudulent activities with remarkable precision.
Transforming the Landscape
The adoption of AI in fraud detection is revolutionizing the insurance industry. By automating complex processes and analyzing data at scale, AI tools offer a new level of insight into claim histories, behavioral patterns, and anomalies that may indicate fraudulent activity.
- Automation of Routine Tasks: AI systems can process and verify large volumes of claims data far more efficiently than human staff.
- Pattern Recognition: Machine learning algorithms excel at identifying subtle patterns that often go unnoticed.
- Real-Time Analysis: Continuous monitoring of claims data enables instant detection of suspicious activities.
These innovations not only reduce the overall cost of fraud but also significantly enhance the speed and accuracy of claims processing. As AI becomes an integral part of fraud detection, insurers can allocate resources more effectively and improve customer satisfaction.
Transitioning from the evolution of AI, we now examine the advanced technologies that are at the forefront of this revolution.
Advanced Technologies Powering AI Fraud Detection
At the core of AI's success in fraud detection are several advanced technologies that work together to create a robust system for identifying anomalies in insurance claims.
Key Technologies and Their Roles
- Machine Learning: Algorithms are trained on historical data to identify the characteristics of fraudulent claims. Over time, they improve their accuracy by learning from new data.
- Predictive Analytics: This technology forecasts future fraudulent activities based on historical trends, allowing insurers to preemptively address potential issues.
- Natural Language Processing (NLP): NLP tools analyze text data from claim forms, emails, and social media to detect inconsistencies or suspicious language patterns.
- Computer Vision: Used for analyzing visual data, computer vision technology can validate the authenticity of submitted photographs or scanned documents.
These technologies are integrated into a unified system that continually evolves as it ingests more data, making fraud detection smarter and more adaptive with each passing day. With such powerful tools in place, the benefits of AI in fraud detection become increasingly apparent.
Benefits of AI in Insurance Fraud Detection
Implementing AI-driven fraud detection systems yields numerous benefits that go beyond simple cost savings. These systems enhance overall operational efficiency and lead to a more secure and trustworthy insurance environment.
Advantages Offered by AI
- Increased Accuracy: AI systems can reduce false positives, ensuring that legitimate claims are not mistakenly flagged, thereby improving customer satisfaction.
- Cost Efficiency: By reducing the number of fraudulent claims processed, insurers can save millions in payouts and administrative costs.
- Faster Processing Times: Automation streamlines the claims process, resulting in quicker resolutions and less downtime for both insurers and policyholders.
- Enhanced Customer Trust: The proactive approach to fraud detection reassures customers that their claims are handled with fairness and transparency.
Insurance companies report that AI-powered systems can cut fraud detection costs by up to 30%, while also increasing claim processing speed and accuracy. With such compelling advantages, it's no surprise that more insurers are integrating AI into their workflows.
As we shift from the benefits to the challenges, it's crucial to understand the hurdles that come with implementing such advanced systems.
Challenges in Implementing AI for Fraud Detection
While AI offers remarkable benefits, its implementation in fraud detection comes with its own set of challenges. Overcoming these obstacles is vital for insurers looking to fully leverage AI technology.
Key Challenges Faced by Insurers
- Data Quality and Quantity: AI systems require large volumes of high-quality data to function optimally. Inconsistent or incomplete data can lead to inaccurate predictions.
- Integration with Legacy Systems: Many insurance companies still rely on outdated systems that are not easily compatible with modern AI solutions.
- Regulatory and Ethical Considerations: Ensuring compliance with privacy laws and maintaining ethical standards in automated decision-making can be complex.
- Skill Gaps: The successful implementation of AI requires a workforce that is adept in both technology and data analysis, which may necessitate additional training or hiring.
Bullet points summarizing major challenges:
- Data Silos: Data is often stored in disparate systems that hinder seamless integration.
- Cost of Implementation: High upfront costs and the need for ongoing maintenance can be a barrier for some insurers.
- Resistance to Change: Organizational inertia and a reluctance to adopt new technologies can slow down implementation efforts.
Navigating these challenges requires a strategic approach and the input of experts. With obstacles in perspective, let’s move into how industry professionals are addressing these issues.
Case Studies and Industry Examples
Real-world case studies illustrate how AI is transforming fraud detection in the insurance sector. These examples provide practical insights into the successful implementation of AI technologies.
Success Stories from the Field
One prominent example involves a major insurer that integrated machine learning into its claims process. The system analyzed historical claim data to create predictive models, leading to a 40% reduction in fraudulent claims within the first year. In another case, a regional insurer used NLP to review claim narratives, significantly reducing the time spent on manual reviews by over 50%.
- Case Study 1: A national insurer reported that AI reduced false positives by 25%, leading to a smoother claims experience for legitimate policyholders.
- Case Study 2: An innovative startup collaborated with an established insurance firm to integrate computer vision technology, enabling rapid verification of submitted evidence and streamlining claim approvals.
These examples demonstrate that AI-driven systems are not only effective but also scalable across different types of insurance providers. As success stories emerge, they pave the way for broader adoption and refinement of AI techniques.
Transitioning from case studies to expert insights, the next section highlights practical tips from professionals in the field.
Expert Opinions and Pro Tips
Industry experts and leading companies offer valuable advice on implementing AI for fraud detection. Their tips underscore the importance of a balanced approach that integrates technology with human oversight.
Key Recommendations from the Experts
- Invest in Quality Data: Experts agree that clean, comprehensive data is the foundation of any successful AI initiative.
- Prioritize Integration: Ensure that AI systems can seamlessly interface with existing technologies and processes.
- Foster a Culture of Innovation: Encourage continuous learning and adaptation among staff to stay ahead of evolving fraud tactics.
- Collaborate with Tech Partners: Working with experienced technology vendors can provide critical support and ensure that AI tools are tailored to specific needs.
A leading industry consultant commented, "The key to a successful AI implementation is not just the technology itself, but the strategic integration of data, processes, and human expertise." These pro tips provide actionable insights for insurers looking to enhance their fraud detection capabilities through AI.
With expert opinions in mind, we now explore how AI integrates with existing systems and workflows.
Integrating AI into Existing Systems
For insurers, the seamless integration of AI into existing systems is crucial for maximizing its benefits. This integration requires thoughtful planning and collaboration across different departments.
Strategies for Effective Integration
- Data Harmonization: Ensure that data from various sources is standardized and accessible for AI algorithms.
- Modular Implementation: Introduce AI tools gradually, starting with pilot projects that can be scaled based on success.
- Continuous Training: Provide ongoing training to staff to ensure they can effectively work with new technologies.
- Collaboration Across Departments: Break down silos between IT, claims, fraud investigation teams, and the use of private investigators to foster a unified approach.
Successful integration of AI systems can streamline operations, reduce manual workloads, and enhance the overall efficiency of fraud detection processes. The focus on integration naturally leads to a discussion of future trends in the field.
Future Trends in AI-Driven Fraud Detection
The evolution of AI continues to shape the future of fraud detection in insurance claims. Emerging trends promise to further enhance the precision and efficiency of these systems.
Emerging Trends to Watch
- Increased Use of Deep Learning: As deep learning models become more sophisticated, they will enable even more accurate predictions and anomaly detection.
- Real-Time Fraud Monitoring: Future systems may offer real-time analysis of claims data, allowing for instantaneous identification and response to fraudulent activities.
- Blockchain Integration: Incorporating blockchain technology could enhance data security and transparency, ensuring that evidence is tamper-proof.
- Augmented Analytics: Combining AI with augmented analytics will empower insurers to visualize complex data sets and gain deeper insights into fraud patterns.
These trends indicate a future where AI not only detects fraud more efficiently but also evolves to address new challenges as they arise. With future trends in focus, let’s now turn to additional strategies for robust fraud prevention.
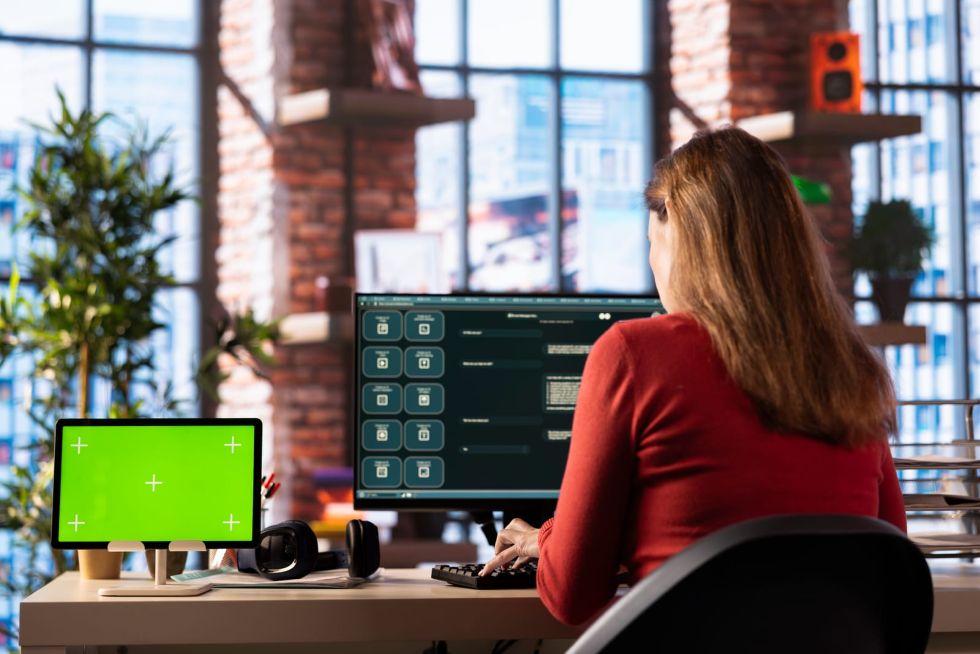
Advanced Analytics for Enhanced Fraud Detection
Beyond the initial detection, advanced analytics plays a critical role in refining and enhancing fraud detection capabilities. These analytics provide a deeper understanding of fraud patterns and help predict future occurrences.
Techniques and Tools
- Behavioral Analysis: Examines the behavior patterns of claimants to identify anomalies that could indicate fraudulent intent.
- Predictive Modeling: Uses historical data to forecast future fraud incidents, enabling proactive measures.
- Anomaly Detection Algorithms: Sophisticated algorithms flag unusual activities, which are then further investigated by human analysts.
- Sentiment Analysis: Analyzes textual data from claim forms and communications to detect inconsistencies in claimant narratives.
These advanced techniques not only support existing fraud detection processes but also add a layer of predictive capability, enhancing overall risk management. With advanced analytics established, the next step is to consider how technology and human expertise work together.
The Synergy of AI and Human Expertise
While AI brings significant advantages to fraud detection, the human element remains indispensable. The synergy between advanced algorithms and human judgment creates a more resilient fraud detection framework.
Balancing Automation and Oversight
- Human Review: AI systems flag potential fraud cases, which are then carefully reviewed by experienced investigators.
- Contextual Interpretation: Humans provide context and intuition that machines may miss, ensuring that decisions are well-rounded.
- Continuous Feedback: Feedback from human analysts helps refine AI algorithms, improving their accuracy over time.
- Collaborative Decision-Making: A partnership between AI and human experts leads to more effective and timely fraud detection.
Insurance companies that integrate human expertise with AI technology have reported improved accuracy and a reduction in false positives, emphasizing the importance of a balanced approach in fraud detection.
Practical Implementation Tips from Industry Leaders
Industry leaders offer actionable insights for insurers embarking on the AI journey for fraud detection. Their experiences provide a roadmap for successful implementation and continuous improvement.
Best Practices for Implementation
- Start Small: Begin with pilot projects to test the effectiveness of AI tools before full-scale implementation.
- Set Clear Metrics: Establish key performance indicators (KPIs) to measure the success of AI initiatives.
- Invest in Training: Ensure that teams are well-versed in both the technological and operational aspects of AI.
- Foster a Culture of Innovation: Encourage continuous learning and adaptation to keep pace with technological advances.
A senior executive at a leading insurance firm noted, "Adopting AI has not only streamlined our fraud detection process but also empowered our teams with the tools to make more informed decisions." These practical tips underscore the importance of a structured and supportive implementation strategy.
Regulatory and Ethical Considerations
As AI becomes integral to fraud detection, regulatory and ethical issues must be addressed to maintain trust and compliance. Insurers must navigate a complex landscape of data privacy laws and ethical standards while implementing cutting-edge technology.
Key Considerations
- Data Privacy: Ensure that all data collection and analysis complies with relevant privacy regulations.
- Transparency: Maintain clear documentation of AI processes to ensure accountability and transparency.
- Bias Mitigation: Regularly audit AI algorithms to prevent unintended biases from affecting outcomes.
- Compliance: Stay updated on changes in regulatory requirements and adjust practices accordingly.
These considerations are essential to building trust with policyholders and regulators alike. With ethical and legal frameworks in place, the next focus is on the overall impact of these systems.
The Impact of AI on the Insurance Industry
The integration of AI into fraud detection has far-reaching implications for the insurance industry. It not only transforms claims processing but also sets new standards for operational efficiency and customer trust.
Industry-Wide Benefits
- Enhanced Efficiency: AI-driven systems reduce processing times and streamline operations.
- Cost Savings: Lower fraud-related payouts lead to substantial savings, which can be passed on to customers.
- Improved Customer Satisfaction: Faster and more accurate claims processing boosts overall customer confidence.
- Market Competitiveness: Insurers that adopt AI technologies gain a competitive edge in the market.
As insurers witness these benefits, the ripple effects are evident throughout the industry, driving innovation and a renewed focus on customer-centric practices.
Summing Up: A New Frontier in Fraud Detection
Artificial intelligence is redefining fraud detection in insurance claims, creating a dynamic landscape where technology and human expertise intersect. The adoption of AI has not only improved the accuracy and efficiency of fraud detection but has also paved the way for innovative solutions that safeguard insurers and their policyholders. Through advanced analytics, machine learning, and cutting-edge technologies, insurers are better equipped than ever to combat fraud, reduce costs, and enhance customer satisfaction.
The transformation brought by AI represents a seismic shift in the way fraud is detected and managed in the insurance industry. With continuous advancements in technology and a collaborative approach that balances automation with human insight, the future of fraud detection looks both promising and exciting. How will emerging technologies further transform the way we fight insurance fraud in the years to come?